You've likely noticed the rising concerns about AI in finance lately. Mike Armstrong, a financial expert, emphasizes the urgent need for tighter data privacy and ethical standards as AI technology advances. With regulatory frameworks struggling to keep up, issues of bias and transparency are surfacing in lending practices. It's crucial to understand how these challenges can impact trust and compliance in the industry. What does this mean for the future of financial operations?
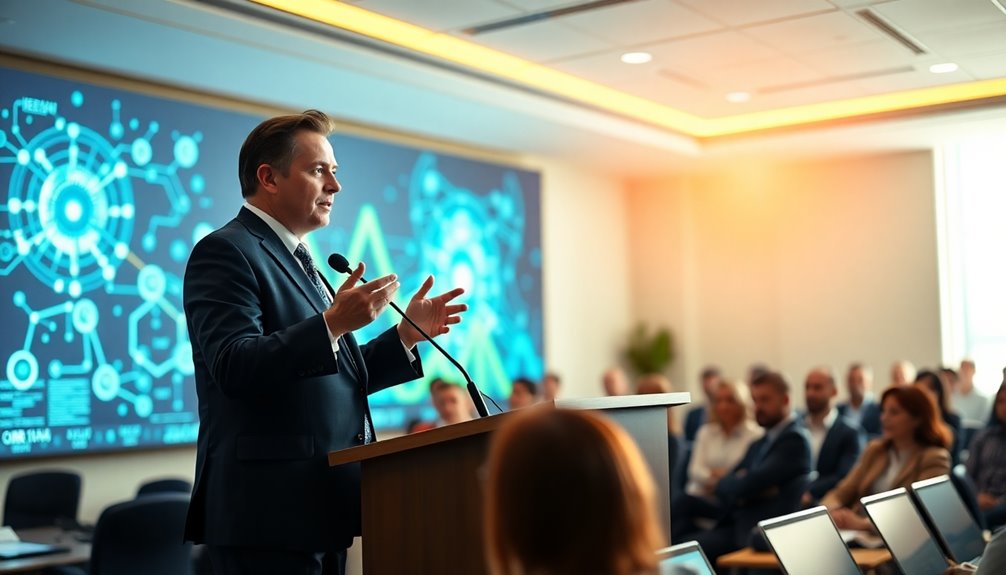
As AI technology rapidly transforms the financial landscape, concerns about data privacy, ethics, and compliance are rising. You might've noticed how AI-driven personalization is reshaping customer experiences in financial services, but with that comes significant privacy concerns. The extensive use of customer data raises red flags, prompting stricter laws around data usage and consent.
As a financial institution, you bear the critical responsibility of preventing data breaches and protecting against fraudulent access. The large datasets that AI relies on expand worries about data protection and compliance, especially since regulations can vary widely across regions.
The ethical implications of AI in finance also warrant your attention. Human-designed algorithms can introduce bias that might skew lending and underwriting processes. If you're not careful, the lack of transparency in your AI models could lead to unfair evaluations of clients, risking regulatory infractions. Mandatory explainable AI in lending decisions will require banks to ensure transparency and fairness in their AI systems.
Regulatory bodies are already demanding transparency in AI credit models to combat discriminatory lending practices. You'll need to ensure that your AI-driven decision-making is fair and ethical; maintaining trust and compliance is essential in today's market. Ignoring these considerations could expose you to legal disputes and damage your firm's reputation.
Operational challenges are another hurdle you'll likely face. The high demand for AI talent in the financial sector drives up hiring costs, making it harder for you to attract skilled professionals. Integrating AI with your existing legacy tech stacks can be cumbersome and expensive, especially in banking.
These outdated systems often limit data quality, which hinders AI's effectiveness. As an IT practitioner, you may find yourself spending considerable time fixing data issues, revealing a gap between what the business expects and the realities of tech integration. Small-scale prototypes can help you identify viable AI use cases, allowing you to mitigate operational risks.
Finally, the regulatory landscape surrounding AI in financial services is constantly evolving. The pace at which AI is being integrated into your operations often outstrips the development of regulations, creating compliance challenges.
Expect regulators to enforce strict transparency and explainability standards for AI algorithms in financial decision-making. As new regulations emerge, ensuring that your AI models are interpretable, fair, and free from bias will be essential for compliance. Given the increasing scrutiny of AI algorithms, particularly in wealth and asset management, staying ahead of these issues will be crucial for your success.